該項目將借助KV260上的PYNQ-DPU覆蓋,從而能夠使我們在LiDAR點云上進行3D對象檢測比以往任何時候都更加高效!
背景
在構建自動駕駛汽車、自動導航機器人和其他現實世界的應用程序時,環境感知起著不可或缺的作用。
為什么要在點云上進行3D對象檢測?
雖然基于深度學習的相機數據二維對象檢測顯示出很高的準確性,但它可能不是有效的活動,如定位、測量對象之間的距離和計算深度信息。
LiDAR傳感器生成的點云提供對象的3D信息,以更有效地定位對象并表征形狀。因此,點云上的3D對象檢測正在各種應用中出現,尤其是在自動駕駛方面。
盡管如此,設計基于LiDAR的3D對象檢測系統仍具有挑戰性。首先,此類系統需要在模型推理中進行大量計算。其次,由于點云數據是不規則的,處理管道需要預處理和后處理才能提供端到端的感知結果。
KV260與3D物體檢測系統完美匹配。模型推理的昂貴計算可以卸載到KV260的可編程邏輯部分并由其加速,而KV260強大的ARM內核能夠處理預處理和后處理任務。
設計概述
我們現在討論選擇的用于點云上3D對象檢測的深度學習模型以及包括軟件和硬件的系統概述。
網絡架構
作為對現有工作的完整性檢查,我們選擇了基于ResNet的關鍵點特征金字塔網絡(KFPN),這是第一個在KITTI基準上具有最先進性能的單目3D檢測實時系統。特別是,我們采用了它在點云上的開源PyTorch實現,稱為SFA3D。
KV260 上的 PYNQ-DPU
之所以我們使用Xilinx 開發板的 Ubuntu Desktop 20.04.3 LTS而不是 Petalinux 作為 KV260 上的操作系統,是因為 Ubuntu 是一個很好的開發環境,用于安裝預處理點云和后處理結果所需的軟件包。另一方面,KV260 對 Pynq 和 DPU 覆蓋的支持避免了從頭設計高效的 DPU,使我們能夠在 python 環境下工作。這在很大程度上簡化了基于 CPU/GPU 的深度學習實現向 KV260 的遷移。
設置環境
按照官方指南將Ubuntu鏡像安裝到KV260,然后參考Github在Ubuntu操作系統中安裝Pynq 。Git 通過執行以下命令克隆所有必需的文件并將所需的包安裝到板上。
git clone https://github.com/SoldierChen/DPU-Accelerated-3D-Object-Detection-on-Point-Clouds.git
cd DPU-Accelerated-3D-Object-Detection-on-Point-Clouds
pip install -r requirements.txt
在這里,我們需要 Pytorch 1.4,因為 Pynq DPU 的 VART 是 v1.4。
數據準備
需要下載的數據包括:
Velodyne 點云(29 GB)
對象數據集的訓練標簽(5 MB)
對象數據集的相機校準矩陣(16 MB)
對象數據集的左側彩色圖像(12 GB) (僅用于可視化目的)
要使用 3D 框可視化 3D 點云,讓我們執行:
cd model_quant_compile/data_process/
python kitti_dataset.py
模型訓練
python train.py --gpu_idx 0
該命令使用一個 GPU 進行訓練,但它支持分布式訓練。此外,您可以選擇 fpn_resnet 或 resnet 作為目標模型。訓練后的模型將存儲在名為“Model_restnet/fpn_resnet_epoch_#”的檢查點文件夾中。根據您的硬件,epoch 可以從 10 到 300,精度越高越好。
模型量化和編譯
同樣,由于 Pynq 的 VART 是 V1.4,我們需要 VITIS AI v1.4 而不是最新版本(V2.0)來進行模型量化。
# install the docker at first (if not stalled)
docker pull xilinx/vitis-ai-cpu:1.4.1.978
# run the docker
./docker_run.sh xilinx/vitis-ai-cpu:1.4.1.978
然后我們使用以下命令量化模型:
# activate the pytorch environment
conda activate vitis-ai-pytorch
# install required packages
pip install -r requirements.txt
# configure the quant_mode to calib
ap.add_argument('-q', '--quant_mode', type=str, default='calib', choices=['calib','test'], help='Quantization mode (calib or test). Default is calib')
# here, it quantize the example model: Model_resnet_18_epoch_10.pth
python quantize.py
# configure the quant_mode to test
ap.add_argument('-q', '--quant_mode', type=str, default='test', choices=['calib','test'], help='Quantization mode (calib or test). Default is calib')
# here, it outputs the quantized model.
python quantize.py
接下來,我們將編譯模型:
./compile.sh zcu102 build/
不要介意 zcu102 與 KV260 共享相同的 DPU 架構。您將看到成功編譯的以下消息:
到目前為止,我們得到了可以在 DPU 上執行的編譯 xmodel,在 KV260 上過度執行。接下來,我們將其部署在板上并開發應用程序代碼。
KV260部署
按照官方指南,我們先在KV260上搭建好Ubuntu操作系統。然后,我們按照PYNQ-DPU GitHub在板上安裝 python 。
搭建好板子后,我們需要安裝git,將代碼克隆到板子上,并將編譯好的xmodel復制到文件夾中。
應用程序代碼設計
這里我們將介紹如何調用并與 DPU 接口進行推理。
我們首先加載 DPU 覆蓋和自定義的 xmodel。然后,重要的是,必須知道輸入和輸出張量信息才能與數據集協調。在這里,我們只有一個張量作為輸入,五個張量作為輸出。相應地分配輸入和輸出緩沖區。
# load model and overly
overlay = DpuOverlay("dpu.bit")
overlay.load_model("./CNN_zcu102.xmodel")
dpu = overlay.runner
# get tensor information
inputTensors = dpu.get_input_tensors()
outputTensors = dpu.get_output_tensors()
shapeIn = tuple(inputTensors[0].dims)
outputSize = int(outputTensors[0].get_data_size() / shapeIn[0])
shapeOut = tuple(outputTensors[0].dims)
shapeOut1 = tuple(outputTensors[1].dims)
shapeOut2 = tuple(outputTensors[2].dims)
shapeOut3 = tuple(outputTensors[3].dims)
shapeOut4 = tuple(outputTensors[4].dims)
# allocate input and output buffers.
# Note the output is a list of five tensors.
output_data = [np.empty(shapeOut, dtype=np.float32, order="C"),
np.empty(shapeOut1, dtype=np.float32, order="C"),
np.empty(shapeOut2, dtype=np.float32, order="C"),
np.empty(shapeOut3, dtype=np.float32, order="C"),
np.empty(shapeOut4, dtype=np.float32, order="C")]
# the input is only one tensor.
input_data = [np.empty(shapeIn, dtype=np.float32, order="C")]
image = input_data[0]
一次性推理的過程封裝在下面的函數中。在這里,我們將輸入張量置換為 DPU 輸入張量的形狀,并將張量置換為后處理所需的形狀。這對于正確的結果至關重要。
def do_detect(dpu, shapeIn, image, input_data, output_data, configs, bevmap, is_front):
if not is_front:
bevmap = torch.flip(bevmap, [1, 2])
input_bev_maps = bevmap.unsqueeze(0).to("cpu", non_blocking=True).float()
# do permutation
input_bev_maps = input_bev_maps.permute(0, 2, 3, 1)
image[0,...] = input_bev_maps[0,...] #.reshape(shapeIn[1:])
job_id = dpu.execute_async(input_data, output_data)
dpu.wait(job_id)
# convert the output arrays to tensors for the following post-processing.
outputs0 = torch.tensor(output_data[0])
outputs1 = torch.tensor(output_data[1])
outputs2 = torch.tensor(output_data[2])
outputs3 = torch.tensor(output_data[3])
outputs4 = torch.tensor(output_data[4])
# do permutation
outputs0 = outputs0.permute(0, 3, 1, 2)
outputs1 = outputs1.permute(0, 3, 1, 2)
outputs2 = outputs2.permute(0, 3, 1, 2)
outputs3 = outputs3.permute(0, 3, 1, 2)
outputs4 = outputs4.permute(0, 3, 1, 2)
outputs0 = _sigmoid(outputs0)
outputs1 = _sigmoid(outputs1)
# post-processing
detections = decode(
outputs0,
outputs1,
outputs2,
outputs3,
outputs4, K=configs.K)
detections = detections.cpu().numpy().astype(np.float32)
detections = post_processing(detections, configs.num_classes, configs.down_ratio, configs.peak_thresh)
return detections[0], bevmap
在 KV260 上執行
通過運行以下命令,將在 DPU 上執行演示數據的推理:
python demo_2_sides-dpu.py
然后運行以下命令:
pythondemo_front-dpu.py
性能范圍從10到20FPS,比在服務器級CPU(IntelXeonGold6226R)上的執行速度快100到200倍。
結論
總之,我們展示了在KV260上使用AMD-XilinxDPU來加速基于點云的3D對象檢測是多么容易。為了進一步提升性能,我們計劃通過使用多個DPU實例來優化模型推理階段,以及通過使用多線程和批處理來優化預處理和后處理階段。
-
3D
+關注
關注
9文章
2862瀏覽量
107324 -
LIDAR
+關注
關注
10文章
323瀏覽量
29357
發布評論請先 登錄
相關推薦
LiDAR如何構建3D點云?如何利用LiDAR提供深度信息
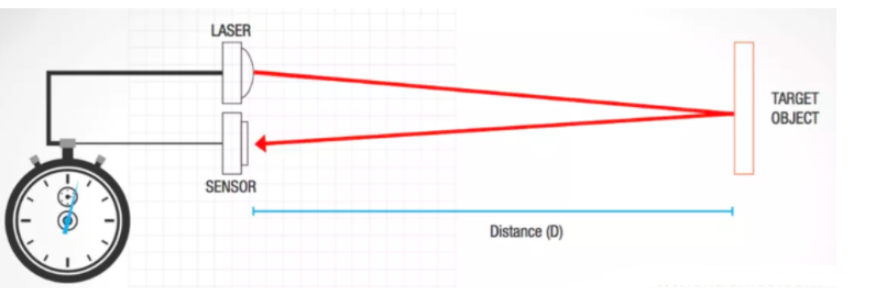
基于深度學習的方法在處理3D點云進行缺陷分類應用
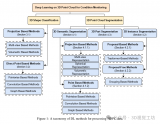
制作3D 對象,然后顯示。 導入3D對象 ,然后顯示。
如何同時獲取2d圖像序列和相應的3d點云?
3D點云技術介紹及其與VR體驗的關系
如何在PADS 3D Layout中進行命令操作
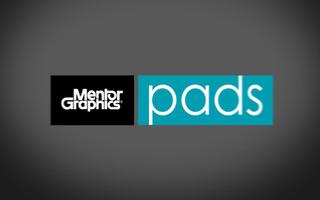
基于圖卷積的層級圖網絡用于基于點云的3D目標檢測
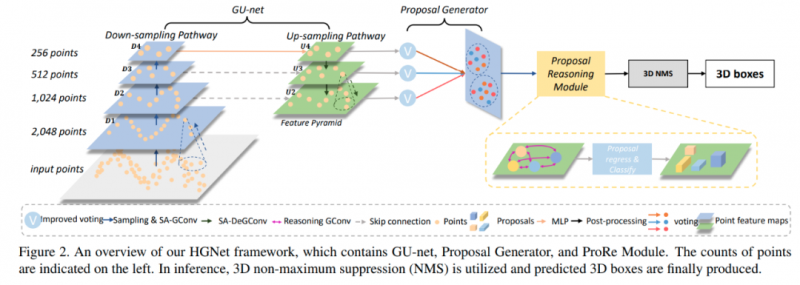
基于層級圖網絡的圖卷積,用點云完成3D目標檢測
3D點云數據集在3D數字化技術中的應用
兩種應用于3D對象檢測的點云深度學習方法
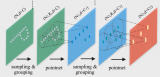
評論