01.使用 BeautifulSoup4 抓取網(wǎng)頁數(shù)據(jù)
所有機(jī)器學(xué)習(xí)(ML)項(xiàng)目的第一步都是收集所需的數(shù)據(jù)。本項(xiàng)目中,我們使用網(wǎng)頁抓取技術(shù)來收集知識(shí)庫數(shù)據(jù)。用 requests
庫獲取網(wǎng)頁并使用 BeautifulSoup4.從網(wǎng)頁中提取信息、解析 HTML 信息并提取段落。
導(dǎo)入 BeautifulSoup4 和 Requests 庫進(jìn)行網(wǎng)頁抓取
運(yùn)行 pip install beautifulsoup4 sentence-transformers
安裝 BeautifulSoup 和 Sentence Transformers。在數(shù)據(jù)抓取部分只需要導(dǎo)入requests和 BeautifulSoup。接下來,創(chuàng)建一個(gè) dictionary,其中包含我們要抓取的 URL 格式。在本示例中,我們只從 Towards Data Science 抓取內(nèi)容,同理也可以從其他網(wǎng)站抓取。
現(xiàn)在,用以下代碼所示的格式從每個(gè)存檔頁面獲取數(shù)據(jù):
import requests
from bs4 import BeautifulSoup
urls = {
'Towards Data Science': '< https://towardsdatascience.com/archive/{0}/{1:02d}/{2:02d} >'
}
此外,我們還需要兩個(gè)輔助函數(shù)來進(jìn)行網(wǎng)頁抓取。第一個(gè)函數(shù)將一年中的天數(shù)轉(zhuǎn)換為月份和日期格式。第二個(gè)函數(shù)從一篇文章中獲取點(diǎn)贊數(shù)。
天數(shù)轉(zhuǎn)換函數(shù)相對(duì)簡單。寫死每個(gè)月的天數(shù),并使用該列表進(jìn)行轉(zhuǎn)換。由于本項(xiàng)目僅抓取 2023 年數(shù)據(jù),因此我們不需要考慮閏年。如果您愿意,可以根據(jù)不同的年份進(jìn)行修改每個(gè)月天數(shù)。
點(diǎn)贊計(jì)數(shù)函數(shù)統(tǒng)計(jì) Medium 上文章的點(diǎn)贊數(shù),單位為 “K” (1K=1000)。因此,在函數(shù)中需要考慮點(diǎn)贊數(shù)中的單位“K”。
def convert_day(day):
month_list = [31, 28, 31, 30, 31, 30, 31, 31, 30, 31, 30, 31]
m = 0
d = 0
while day > 0:
m += 1
d = day
day -= month_list[m-1]
return (m, d)
def get_claps(claps_str):
if (claps_str is None) or (claps_str == '') or (claps_str.split is None):
return 0
split = claps_str.split('K')
claps = float(split[0])
return int(claps*1000) if len(split) == 2 else int(claps)
解析 BeautifulSoup4 的網(wǎng)頁抓取響應(yīng)
現(xiàn)在已經(jīng)設(shè)置好必要的組件,可以進(jìn)行網(wǎng)頁抓取。為了避免在過程中遇到 429 錯(cuò)誤(請(qǐng)求過多),我們使用 time 庫,在發(fā)送請(qǐng)求之間引入延遲。此外,用 sentence transformers 庫從 Hugging Face 獲取 embedding 模型—— MiniLM 模型。
如前所述,我們只抓取了 2023 年的數(shù)據(jù),所以將年份設(shè)置為 2023。此外,只需要從第 1 天(1 月 1 日)到第 244 天(8 月 31 日)的數(shù)據(jù)。根據(jù)設(shè)定的天數(shù)進(jìn)行循環(huán),每個(gè)循環(huán)在第一次調(diào)用time.sleep()
之前會(huì)首先設(shè)置必要的組件。我們會(huì)把天數(shù)轉(zhuǎn)換成月份和日期,并轉(zhuǎn)成字符串,然后根據(jù) urls 字典組成完整的 URL,最后發(fā)送請(qǐng)求獲取 HTML 響應(yīng)。
獲取 HTML 響應(yīng)之后,使用 BeautifulSoup 進(jìn)行解析,并搜索具有特定類名(在代碼中指示)的div
元素,該類名表示它是一篇文章。我們從中解析標(biāo)題、副標(biāo)題、文章 URL、點(diǎn)贊數(shù)、閱讀時(shí)長和回應(yīng)數(shù)。隨后,再次使用requests
來獲取文章的內(nèi)容。每次通過請(qǐng)求獲取文章內(nèi)容后,都會(huì)再次調(diào)用time.sleep()
。此時(shí),我們已經(jīng)獲取了大部分所需的文章元數(shù)據(jù)。提取文章的每個(gè)段落,并使用我們的 HuggingFace 模型獲得對(duì)應(yīng)的向量。接著,創(chuàng)建一個(gè)字典包含該文章段落的所有元信息。
import time
from sentence_transformers import SentenceTransformer
model = SentenceTransformer("sentence-transformers/all-MiniLM-L12-v2")
data_batch = []
year = 2023
for i in range(1, 243):
month, day = convert_day(i)
date = '{0}-{1:02d}-{2:02d}'.format(year, month, day)
for publication, url in urls.items():
response = requests.get(url.format(year, month, day), allow_redirects=True)
if not response.url.startswith(url.format(year, month, day)):
continue
time.sleep(8)
soup = BeautifulSoup(response.content, 'html.parser')
articles = soup.find_all("div","postArticle postArticle--short js-postArticle js-trackPostPresentation js-trackPostScrolls")
for article in articles:
title = article.find("h3", class_="graf--title")
if title is None:
continue
title = str(title.contents[0]).replace(u'\xA0', u' ').replace(u'\u200a', u' ')
subtitle = article.find("h4", class_="graf--subtitle")
subtitle = str(subtitle.contents[0]).replace(u'\xA0', u' ').replace(u'\u200a', u' ') if subtitle is not None else ''
article_url = article.find_all("a")[3]['href'].split('?')[0]
claps = get_claps(article.find_all("button")[1].contents[0])
reading_time = article.find("span", class_="readingTime")
reading_time = int(reading_time['title'].split(' ')[0]) if reading_time is not None else 0
responses = article.find_all("a", class_="button")
responses = int(responses[6].contents[0].split(' ')[0]) if len(responses) == 7 else (0 if len(responses) == 0 else int(responses[0].contents[0].split(' ')[0]))
article_res = requests.get(article_url)
time.sleep(8)
paragraphs = BeautifulSoup(article_res.content, 'html.parser').find_all("[class*="pw-post-body-paragraph"]")
for i, paragraph in enumerate(paragraphs):
embedding = model.encode([paragraph.text])[0].tolist()
data_batch.append({
"_id": f"{article_url}+{i}",
"article_url": article_url,
"title": title,
"subtitle": subtitle,
"claps": claps,
"responses": responses,
"reading_time": reading_time,
"publication": publication,
"date": date,
"paragraph": paragraph.text,
"embedding": embedding
})
最后一步是使用 pickle 處理文件。
filename = "TDS_8_30_2023"
with open(f'{filename}.pkl', 'wb') as f:
pickle.dump(data_batch, f)
數(shù)據(jù)呈現(xiàn)
數(shù)據(jù)可視化十分有用。下面是在 Zilliz Cloud 中數(shù)據(jù)的樣子。請(qǐng)注意其中的 embedding,這些數(shù)據(jù)表示了文檔向量,也就是我們根據(jù)文章段落生成的向量。
02.將 TDS 數(shù)據(jù)導(dǎo)入到向量數(shù)據(jù)庫中
獲取數(shù)據(jù)后,下一步是將其導(dǎo)入到向量數(shù)據(jù)庫中。在本項(xiàng)目中,我們使用了一個(gè)單獨(dú)的 notebook 將數(shù)據(jù)導(dǎo)入到 Zilliz Cloud,而不是從 Towards Data Science 進(jìn)行網(wǎng)頁抓取。
要將數(shù)據(jù)插入 Zilliz Cloud,需按照以下步驟進(jìn)行操作:
- 連接到 Zilliz Cloud
- 定義 Collection 的參數(shù)
- 將數(shù)據(jù)插入 Zilliz Cloud
設(shè)置 Jupyter Notebook
運(yùn)行 pip install pymilvus python-dotenv
來設(shè)置 Jupyter Notebook 并啟動(dòng)數(shù)據(jù)導(dǎo)入過程。用 dotenv
庫來管理環(huán)境變量。對(duì)于pymilvus
包,需要導(dǎo)入以下模塊:
utility
用于檢查集合的狀態(tài)connections
用于連接到 Milvus 實(shí)例FieldSchema
用于定義字段的 schemaCollectionSchema
用于定義 collection schemaDataType
字段中存儲(chǔ)的數(shù)據(jù)類型Collection
我們?cè)L問 collection 的方式
然后,打開之前 pickle 的數(shù)據(jù),獲取環(huán)境變量,并連接到 Zilliz Cloud。
import pickle
import os
from dotenv import load_dotenv
from pymilvus import utility, connections, FieldSchema, CollectionSchema, DataType, Collection
filename="TDS_8_30_2023"
with open(f'{filename}.pkl', 'rb') as f:
data_batch = pickle.load(f)
zilliz_uri = "your_zilliz_uri"
zilliz_token = "your_zilliz_token"
connections.connect(
uri= zilliz_uri,
token= zilliz_token
)
設(shè)置 Zilliz Cloud 向量數(shù)據(jù)庫并導(dǎo)入數(shù)據(jù)
接下來,需要設(shè)置 Zilliz Cloud。我們必須創(chuàng)建一個(gè) Collection 來存儲(chǔ)和組織從 TDS 網(wǎng)站抓取的數(shù)據(jù)。需要兩個(gè)常量:dimension(維度)和 collection name(集合名稱),dimension 是指我們的向量具有的維度數(shù)。在本項(xiàng)目中,我們使用 384 維的 MiniLM 模型。
Milvus 的全新 Dynamic schema 功能允許我們僅為 Collection 設(shè)置 ID 和向量字段,無需考慮其他字段數(shù)量和數(shù)據(jù)類型。注意,需要記住保存的特定字段名稱,因?yàn)檫@對(duì)于正確檢索字段至關(guān)重要。
DIMENSION=384
COLLECTION_NAME="tds_articles"
fields = [
FieldSchema(name='id', dtype=DataType.VARCHAR, max_length=200, is_primary=True),
FieldSchema(name='embedding', dtype=DataType.FLOAT_VECTOR, dim=DIMENSION)
]
schema = CollectionSchema(fields=fields, enable_dynamic_field=True)
collection = Collection(name=COLLECTION_NAME, schema=schema)
index_params = {
"index_type": "AUTO_INDEX",
"metric_type": "L2",
"params": {"nlist": 128},
}
collection.create_index(field_name="embedding", index_params=index_params)
Collection 有兩種插入數(shù)據(jù)的選項(xiàng):
- 遍歷數(shù)據(jù)并逐個(gè)插入每個(gè)數(shù)據(jù)
- 批量插入數(shù)據(jù)
在插入所有數(shù)據(jù)之后,重要的是刷新集合以進(jìn)行索引并確保一致性,導(dǎo)入大量數(shù)據(jù)可能需要一些時(shí)間。
for data in data_batch:
collection.insert([data])
collection.flush()
03.查詢 TDS 文章片段
一切準(zhǔn)備就緒后,就可以進(jìn)行查詢了。
獲取 HuggingFace 模型并設(shè)置 Zilliz Cloud 查詢
注意,必須獲取 embedding 模型并設(shè)置向量數(shù)據(jù)庫以查詢 Towards Data Science 知識(shí)庫。這一步使用了一個(gè)單獨(dú)的筆記本。我們將使用dotenv
庫來管理環(huán)境變量。此外,還需要使用 Sentence Transformers 中的 MiniLM 模型。這一步中,可以重用 Web Scraping 部分提供的代碼。
import os
from dotenv import load_dotenv
from pymilvus import connections, Collection
zilliz_uri = "your_zilliz_uri"
zilliz_token = "your_zilliz_token"
from sentence_transformers import SentenceTransformer
model = SentenceTransformer("sentence-transformers/all-MiniLM-L12-v2")
執(zhí)行向量搜索查詢
連接到向量數(shù)據(jù)庫并執(zhí)行搜索。在本項(xiàng)目中,我們將連接到一個(gè) Zilliz Cloud 實(shí)例,并檢索之前創(chuàng)建的集合 tds_articles
,用戶要先輸入他們的查詢問題。
接下來,使用 Hugging Face 的 embedding 模型對(duì)查詢進(jìn)行編碼。這個(gè)過程將用戶的問題轉(zhuǎn)換為一個(gè) 384 維的向量。然后,使用這個(gè)編碼后的查詢向量來搜索向量數(shù)據(jù)庫。在搜索過程中,需要指定進(jìn)行 ANN 查詢字段(anns_field
)、索引參數(shù)、期望的搜索結(jié)果數(shù)量限制以及我們想要的輸出字段(output fields)。
之前,我們用了 Milvus 的 Dynamic Schema 特性來簡化字段 Schema 定義流程。搜索向量數(shù)據(jù)庫時(shí),包括所需的動(dòng)態(tài)字段在搜索結(jié)果中是必要的。這個(gè)特定的場景涉及請(qǐng)求paragraph
字段,其中包含文章中每個(gè)段落的文本。
connections.connect(uri=zilliz_uri, token=zilliz_token)
collection = Collection(name="tds_articles")
query = input("What would you like to ask Towards Data Science's 2023 publications up to September? ")
embedding = model.encode(query)
closest = collection.search([embedding],
anns_field='embedding',
param={"metric_type": "L2",
"params": {"nprobe": 16}},
limit=2,
output_fields=["paragraph"])
print(closest[0][0])
print(closest[0][1])
比如,我在應(yīng)用中查詢大語言模型相關(guān)的信息,返回了以下兩個(gè)回答。盡管這些回答提到了“語言模型”并包含一些相關(guān)信息,但它們沒有提供關(guān)于大型語言模型的詳細(xì)解釋。第二個(gè)回答在語義上相似,但是不足夠接近我們想要的內(nèi)容。
04.給向量數(shù)據(jù)庫知識(shí)庫添加內(nèi)容
到目前為止,我們使用 Zilliz Cloud 作為向量數(shù)據(jù)庫在 TDS 文章上創(chuàng)建了一個(gè)知識(shí)庫。雖然能夠輕松地檢索語義上相似的搜索結(jié)果,但還沒有達(dá)到我們的期望。下一步是通過加入新的框架和技術(shù)來增強(qiáng)我們的結(jié)果。
05.總結(jié)
本教程介紹了如何基于 Towards Data Science 文章構(gòu)建聊天機(jī)器人。我們演示了網(wǎng)頁爬取的過程,創(chuàng)建了知識(shí)庫,包括將文本轉(zhuǎn)換成向量存儲(chǔ)在 Zilliz Cloud 中。然后,我們演示了如何提示用戶進(jìn)行查詢,將查詢轉(zhuǎn)化為向量,并查詢向量數(shù)據(jù)庫。
不過,雖然結(jié)果在語義上相似,但并不完全符合我們的期望。在本系列的下一篇中,我們將探討使用 LlamaIndex 來優(yōu)化查詢。除了這里討論的步驟之外,大家也可以結(jié)合 Zilliz Cloud 嘗試替換模型、合并文本或使用其他數(shù)據(jù)集。
-
編碼器
+關(guān)注
關(guān)注
44文章
3506瀏覽量
132913 -
URL
+關(guān)注
關(guān)注
0文章
138瀏覽量
15142 -
機(jī)器學(xué)習(xí)
+關(guān)注
關(guān)注
66文章
8293瀏覽量
131683 -
TDS
+關(guān)注
關(guān)注
0文章
14瀏覽量
14354 -
聊天機(jī)器人
+關(guān)注
關(guān)注
0文章
323瀏覽量
12250
發(fā)布評(píng)論請(qǐng)先 登錄
相關(guān)推薦
聊天機(jī)器人+微信平臺(tái)檢測(cè)環(huán)境,方案分享啦
聊天機(jī)器人在國內(nèi)為什么只能做客服?
聊天機(jī)器人的自動(dòng)問答技術(shù)實(shí)現(xiàn)
如何利用Python+ESP8266 DIY 一個(gè)智能聊天機(jī)器人?
五步詮釋聊天機(jī)器人
聊天機(jī)器人的作用分析
如何避免聊天機(jī)器人的5個(gè)錯(cuò)誤策略
一個(gè)基于Microsoft聊天機(jī)器人Tay的示例
智能聊天機(jī)器人的會(huì)話式應(yīng)用平臺(tái)日趨成熟化
構(gòu)建聊天機(jī)器人需要哪些資源?
GoGlobal 推出全新 AI 聊天機(jī)器人 – ChatGoGlobal
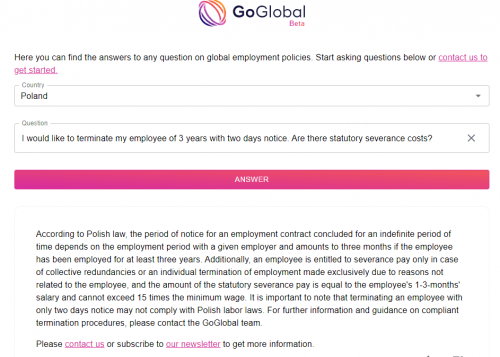
評(píng)論