作者:
顏國進 英特爾邊緣計算創新大使
2024英特爾“走近開發者”互動活動-哪吒開發套件免費試 用 AI 創新計劃:哪吒開發板是專為支持入門級邊緣 AI 應用程序和設備而設計,能夠滿足人工智能學習、開發、實訓等應用場景。為了測試該開發板的推理性能,同時測試所推出的 OpenVINO C# API (https://github.com/guojin-yan/OpenVINO-CSharp-API) 項目能否應用到該開發板上,我們使用該開發板,結合 OpenVINO C# API 的異步推理功能,加速深度學習推理速度。
1哪吒開發板
1.1 產品簡介
哪吒(Nezha)開發套件以信用卡大小(85 x 56mm)的開發板-哪吒(Nezha)為核心,哪吒采用Intel N97處理器(Alder Lake-N),最大睿頻 3.6GHz,Intel UHD Graphics 內核 GPU,可實現高分辨率顯示;板載 LPDDR5 內存、eMMC 存儲及 TPM 2.0,配備 GPIO 接口,支持 Windows 和 Linux 操作系統,這些功能和無風扇散熱方式相結合,為各種應用程序構建高效的解決方案,適用于如自動化、物聯網網關、數字標牌和機器人等應用。
該開發板是類樹莓派的 x86 主機,可支持 Linux Ubuntu 及完整版 Windows 操作系統。板載英特爾 N97 處理器,最高運行頻率可達 3.6 GHz,且內置顯卡(iGPU),板載 64GB eMMC 存儲及 LPDDR5 4800MHz(4GB/8GB),支持 USB 3.0、HDMI 視頻輸出、3.5mm 音頻接口、1000Mbps 以太網口。完全可把它作為一臺 mini 小電腦來看待,且其可外接 Arduino,STM32 等單片機,擴展更多應用及各種傳感器模塊。
此外, 其主要接口與 Jetson Nano 載板兼容,GPIO 與樹莓派兼容,能夠最大限度地復用樹莓派、Jetson Nano 等生態資源,無論是自動化、物聯網網關、數字標牌或是攝像頭物體識別、3D 打印,還是 CNC 實時插補控制都能穩定運行。可作為邊緣計算引擎用于人工智能產品驗證、開發;也可以作為域控核心用于機器人產品開發。
1.2 功能特點
Intel Processor N97
板載 LPDDR5 內存, 8GB
板載 eMMC 存儲, 64GB
1Gigabit LAN x 1
HDMI 1.4b x 1
USB 3.2 Gen 2 (Type-A) x 3, 10針 USB 2.0 x 2/UART x 1
40針 GPIO x 1
12V 直流輸入, 5A
TPM 2.0
下圖為哪吒開發板產品介紹圖片:
2環境配置
2.1NET 8.0 安裝
進入微軟官網下載地址:
https://dotnet.microsoft.com/zh-cn/download
顯示如下頁面直接下載即可:
下載完成過后,右擊以管理員方式運行即可,進入一下頁面后,直接點擊安裝,按照默認方式安裝即可:
安裝完成后,在 CMD 中輸入 dotnet --info 指令,查看是否安裝成功:
2.2VS Code 配置 C# 環境
VS Code 安裝較為簡單,大家直接網上下載安裝包安裝即可,此處不做太多的贅述。進入 VS Code,在擴展中,依次安裝一下插件:
安裝完成后,便可以進行 C# 項目編輯。
3創建并配置 YOLOv8 推理項目
3.1創建 YOLOv8 推理項目
此處我們使用 CMD 創建項目,首先輸入以下指令:
dotnet new console -o yolov8_async_csharp -f net8.0
輸入指令后,結果如下圖所示:
3.2配置 YOLOv8 推理項目
該項目中需要配置 OpenCV 和 OpenVINO 依賴,其中 OpenCV 我們在 C# 使用的是 OpenCvSharp4,而 OpenVINO 就是使用的我們開發的[OpenVINO C# API] (https://github.com/guojin-yan/OpenVINO-CSharp-API) 項目,該項目均可以通過 NUGET 安裝,在該項目中,輸入以下指令,進行以來安裝:
OpenCvSharp4 安裝指令:
dotnet add package OpenCvSharp4 --version 4.9.0.20240103 dotnet add package OpenCvSharp4.runtime.win --version 4.9.0.20240103
OpenVINO C# API 安裝指令:
dotnet add package OpenVINO.CSharp.API --version 2024.3.0.2 dotnet add package OpenVINO.runtime.win --version 2024.3.0.1 dotnet add package OpenVINO.CSharp.API.Extensions.OpenCvSharp --version 1.0.6.1
4編寫推理代碼
下面為該項目所使用的所有代碼,為了提高推理速度,此處使用的為異步推理過程。
using OpenCvSharp.Dnn; using OpenCvSharp; using OpenVinoSharp; using OpenVinoSharp.Extensions.result; using OpenVinoSharp.Extensions.process; using System.Diagnostics; using OpenVinoSharp.preprocess; namespace openvino_async_csharp { internal class Program { static void Main(string[] args) { Console.WriteLine("Hello, World!"); yolov8_async_det(); } static void yolov8_async_det() { string video_path = "video.mp4"; string model_path = "yolov8s.onnx"; Core core = new Core(); Model model = core.read_model(model_path); CompiledModel compiled_model = core.compile_model(model, "GPU.0"); VideoCapture capture = new VideoCapture(video_path); if (!capture.IsOpened()) { Console.WriteLine("ERROR: 視頻無法打開"); return; } Listrequests = new List { compiled_model.create_infer_request(), compiled_model.create_infer_request() }; Mat frame = new Mat(); capture.Read(frame); float factor = 0f; float[] input_data = preprocess(frame, out factor); requests[0].get_input_tensor().set_data(input_data); requests[0].start_async(); Stopwatch sw = new Stopwatch(); float[] total_infs = new float[3]; while (true) { Mat next_frame = new Mat(); if (!capture.Read(next_frame)) { break; } sw.Restart(); input_data = preprocess(frame, out factor); requests[1].get_input_tensor().set_data(input_data); sw.Stop(); total_infs[0] = sw.ElapsedMilliseconds; sw.Restart(); requests[1].start_async(); requests[0].wait(); sw.Stop(); total_infs[1] = sw.ElapsedMilliseconds; sw.Restart(); float[] output_data = requests[0].get_output_tensor().get_data (8400 * 84); DetResult result = postprocess(output_data, factor); sw.Stop(); total_infs[2] = sw.ElapsedMilliseconds; Cv2.PutText(frame, "PreProcess: " + (1000.0 / total_infs[0]).ToString("0.00") + "FPS " + (total_infs[0]).ToString("0.00") + "ms", new Point(20, 40), HersheyFonts.HersheyPlain, 2, new Scalar(255, 0, 255), 2); Cv2.PutText(frame, "Inference: " + (1000.0 / total_infs[1]).ToString("0.00") + "FPS " + (total_infs[1]).ToString("0.00") + "ms", new Point(20, 70), HersheyFonts.HersheyPlain, 2, new Scalar(255, 0, 255), 2); Cv2.PutText(frame, "PostProcess: " + (1000.0 / total_infs[2]).ToString("0.00") + "FPS " + (total_infs[2]).ToString("0.00") + "ms", new Point(20, 100), HersheyFonts.HersheyPlain, 2, new Scalar(255, 0, 255), 2); Cv2.PutText(frame, "Total: " + (1000.0 / (total_infs[0] + total_infs[1] + total_infs[2])).ToString("0.00") + "FPS " + ((total_infs[0] + total_infs[1] + total_infs[2])).ToString("0.00") + "ms", new Point(20, 130), HersheyFonts.HersheyPlain, 2, new Scalar(255, 0, 255), 2); Mat res_mat = Visualize.draw_det_result(result, frame); Cv2.ImShow("Result", res_mat); Cv2.WaitKey(10); swap(requests); frame = next_frame; } } public static float[] preprocess(Mat img, out float factor) { Mat mat = new Mat(); Cv2.CvtColor(img, mat, ColorConversionCodes.BGR2RGB); mat = Resize.letterbox_img(mat, 640, out factor); mat = Normalize.run(mat, true); return Permute.run(mat); } public static DetResult postprocess(float[] result, float factor) { // Storage results list List positionBoxes = new List (); List classIds = new List (); List confidences = new List (); // Preprocessing output results for (int i = 0; i < 8400; i++) ? ? ? ? ?{ ? ? ? ? ? ? ? for (int j = 4; j < 84; j++) ? ? ? ? ? ? ?{ ? ? ? ? ? ? ? ? ? float source = result[8400 * j + i]; ? ? ? ? ? ? ? ? ? int label = j - 4; ? ? ? ? ? ? ? ? ? if (source > 0.2) { float maxSource = source; float cx = result[8400 * 0 + i]; float cy = result[8400 * 1 + i]; float ow = result[8400 * 2 + i]; float oh = result[8400 * 3 + i]; int x = (int)((cx - 0.5 * ow) * factor); int y = (int)((cy - 0.5 * oh) * factor); int width = (int)(ow * factor); int height = (int)(oh * factor); Rect box = new Rect(x, y, width, height); positionBoxes.Add(box); classIds.Add(label); confidences.Add(maxSource); } } } DetResult re = new DetResult(); int[] indexes = new int[positionBoxes.Count]; CvDnn.NMSBoxes(positionBoxes, confidences, 0.2f, 0.5f, out indexes); for (int i = 0; i < indexes.Length; i++) ? ? ? ? ?{ ? ? ? ? ? ? ? int index = indexes[i]; ? ? ? ? ? ? ? re.add(classIds[index], confidences[index], positionBoxes[index]); ? ? ? ? ?} ? ? ? ? ? return re; ? ? ?} ? ? ? public static void swap(List requests) { //(requests[0], requests[1]) = (requests[1], requests[0]); var tmp = requests[0]; requests[0] = requests[1]; requests[1] = tmp; } } }
打開創建的項目,編輯 Program.cs 文件,將上述代碼替換該文件中的代碼即可。
5項目編譯與運行
輸入以下指令后進行項目編譯:
dotnet build --configuration Release
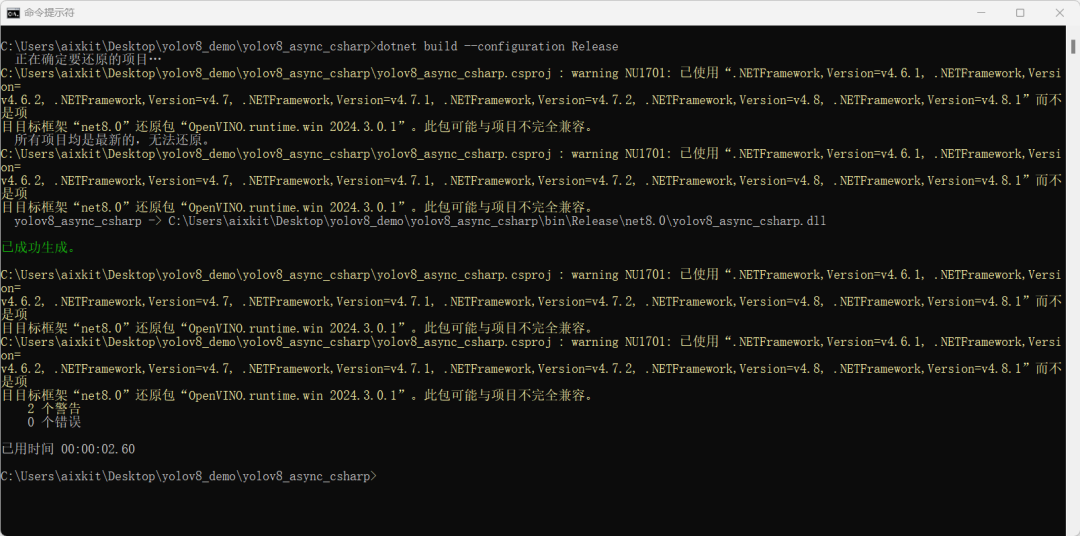
通過上圖可以看出,該項目編譯成功,未出現任何問題,接下來輸入以下指令進行項目運行:
dotnet run --configuration Release
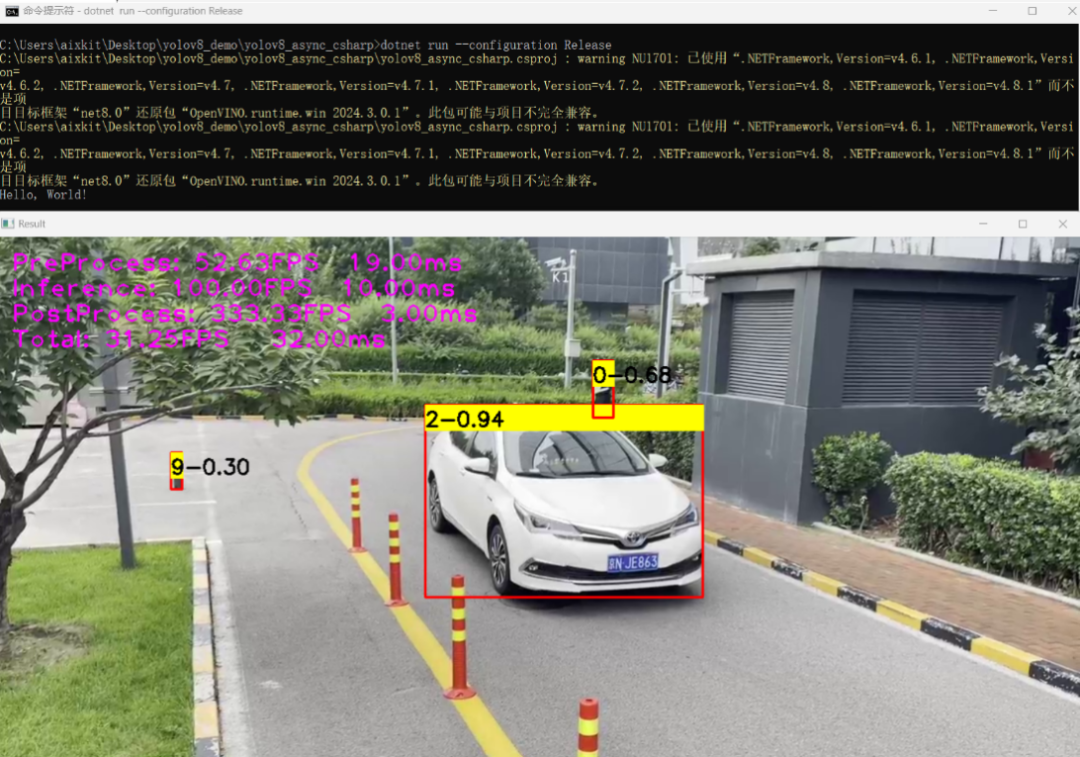
上圖為運行后的輸出和推理效果,可以看出使用異步推理后,可以實現20幀以上的推理速度。
為了對比推理效果,此處還開展了同步推理實現,異步推理速度可以實現到 25FPS 以上,而同步推理只能達到 12FPS 左右,其推理速度提升了一倍,且滿足視頻的實時推理要求。
6總結
在該項目中,我們使用哪吒開發板套件,通過前期推出的 OpenVINO C# API 項目,實現了在開發板環境下部署 YOLOv8 模型,并進行了視頻推理測試。在使用哪吒開發板套件的 IGPU 設備進行推理時,其推理速度平均可以達到 25FPS 以上,可以實現視頻實時推理。
-
英特爾
+關注
關注
60文章
9880瀏覽量
171479 -
接口
+關注
關注
33文章
8496瀏覽量
150829 -
AI
+關注
關注
87文章
30106瀏覽量
268398 -
開發板
+關注
關注
25文章
4943瀏覽量
97188 -
OpenVINO
+關注
關注
0文章
87瀏覽量
181
原文標題:開發者實戰|使用 OpenVINO? C# 異步推理接口部署 YOLOv8 ——在哪吒開發板上也可以實現視頻推理
文章出處:【微信號:英特爾物聯網,微信公眾號:英特爾物聯網】歡迎添加關注!文章轉載請注明出處。
發布評論請先 登錄
相關推薦
【愛芯派 Pro 開發板試用體驗】yolov8模型轉換
TensorRT 8.6 C++開發環境配置與YOLOv8實例分割推理演示
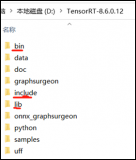
在AI愛克斯開發板上用OpenVINO?加速YOLOv8目標檢測模型
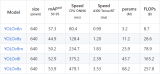
在AI愛克斯開發板上用OpenVINO?加速YOLOv8-seg實例分割模型
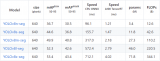
教你如何用兩行代碼搞定YOLOv8各種模型推理
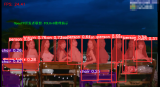
在AI愛克斯開發板上用OpenVINO?加速YOLOv8-seg實例分割模型
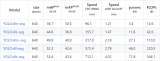
三種主流模型部署框架YOLOv8推理演示
基于YOLOv8的自定義醫學圖像分割
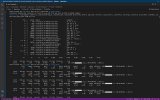
基于OpenCV DNN實現YOLOv8的模型部署與推理演示
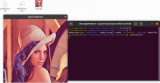
評論