前段時(shí)間TF發(fā)布了2.0版本,今天略讀了一番,發(fā)現(xiàn)新特性確實(shí)可以在開(kāi)發(fā)中節(jié)省很多時(shí)間。閱讀的同時(shí),順便將開(kāi)發(fā)指南翻譯了一點(diǎn),如有錯(cuò)誤歡迎指正。
Effective TensorFlow 2.0
為使TensorFLow用戶更高效,TensorFlow 2.0中進(jìn)行了多出更改。TensorFlow 2.0刪除了篇冗余API,使API更加一致(統(tǒng)一RNNs, 統(tǒng)一優(yōu)化器),并通過(guò)Eager execution更好地與Python集成。
許多RFCs已經(jīng)解釋了TensorFlow 2.0帶來(lái)的變化。本指南介紹了TensorFlow 2.0應(yīng)該怎么進(jìn)行開(kāi)發(fā)。這假設(shè)您已對(duì)TensorFlow 1.x有一定了解。
A brief summary of major changes
API Cleanup
許多API在TF 2.0中進(jìn)行了移動(dòng)或刪除。一些主要的變化包括刪除tf.app,tf.flags,使tf.logging支持現(xiàn)在開(kāi)源的absl-py,重新生成項(xiàng)目的tf.contribe,通過(guò)清理tf.*中那些較少使用的命名空間,例如tf.math。一些API已替換為自己的2.0版本-tf.summary,tf.keras.metrics, 和tf.keras.optimizers。最快升級(jí)應(yīng)用這些重命名帶來(lái)的變化可使用v2升級(jí)腳本。
Eager execution
TensorFlow 1.x要求用戶通過(guò)tf.*API手動(dòng)的將抽象語(yǔ)法樹(shù)(圖)拼接在一起。然后它要求用戶通過(guò)一組輸入、輸出張量傳遞給session.run()從而手動(dòng)編譯調(diào)用這個(gè)圖。TensorFlow 2.0 Eager execution可以像Python那樣執(zhí)行,在2.0中,graph 和 session會(huì)像實(shí)現(xiàn)細(xì)節(jié)一樣。
值得注意的是tf.control_dependencies()不再需要了,因?yàn)樗写a都是行順序執(zhí)行的(用tf.function聲明)。
No more globals
TensorFlow 1.x嚴(yán)重依賴隱式全局命名空間。當(dāng)你調(diào)用tf.Variable(),它會(huì)被放入默認(rèn)圖中,即使你忘了指向它的Python變量,它也會(huì)被保留在那里。然后你可以恢復(fù)它,但前提是你得知道它創(chuàng)建時(shí)的名稱。如果你無(wú)法控制變量的創(chuàng)建,這很難做到。其結(jié)果是,各種各樣的機(jī)制,試圖幫助用戶再次找到他們的變量,以及為框架找到用戶創(chuàng)建的變量:Variable scopes, global collections。例如tf.get_global_step(),tf.global_variables_initializer(),還有優(yōu)化器隱式計(jì)算所有可訓(xùn)練變量的梯度等等。TensorFlow 2.0消除了這些機(jī)制(Variable 2.0 RFC)默認(rèn)支持的機(jī)制:跟蹤你的變量!如果你忘記了一個(gè)tf.Variable,它就會(huì)當(dāng)作垃圾被回收。
Functions, not sessions
session.run()幾乎可以像函數(shù)一樣調(diào)用:指定輸入和被調(diào)用的函數(shù),你可以得到一組輸出。在TensorFlow 2.0中,您可以使用Python函數(shù)tf.function()來(lái)標(biāo)記它以進(jìn)行JIT編譯,以便TensorFlow將其作為單個(gè)圖運(yùn)行(Function 2.0 RFC)。這種機(jī)制允許TensorFlow 2.0獲得圖模型所有的好處:
性能:函數(shù)可以被優(yōu)化(node pruning, kernel fusion, etc.)
可移植性:該功能可以被導(dǎo)出/重新導(dǎo)入(SavedModel 2.0 RFC),允許用戶重用和共享模塊化TensorFlow功能。
# TensorFlow 1.X
outputs = session.run(f(placeholder), feed_dict={placeholder: input})
# TensorFlow 2.0
outputs = f(input)
憑借穿插Python 和TensorFlow代碼的能力,我們希望用戶能夠充分利用Python的表現(xiàn)力。除了在沒(méi)有Python解釋器的情況下執(zhí)行TensorFlow,如mobile, C++, 和 JS。為了幫助用戶避免在添加時(shí)重寫(xiě)代碼@tf.function, AutoGraph會(huì)將Python構(gòu)造的一個(gè)子集轉(zhuǎn)換為他們的TensorFlow等價(jià)物:
for/while -> tf.while_loop (支持break 和 continue)
if->tf.cond
for _ in dataset -> dataset.reduce
AutoGraph支持控制流的任意嵌套,這使得可以有較好性能并且簡(jiǎn)潔地實(shí)現(xiàn)許多復(fù)雜的ML程序,如序列模型,強(qiáng)化學(xué)習(xí),自定義訓(xùn)練循環(huán)等。
Recommendations for idiomatic TensorFlow 2.0
Refactor your code into smaller functions
TensorFlow 1.x中常見(jiàn)使用模式是“kitchen sink”策略,其中所有可能的計(jì)算的聯(lián)合被預(yù)先布置,然后選擇被評(píng)估的張量,通過(guò)session.run()運(yùn)行。在TensorFlow 2.0中,用戶應(yīng)該將代碼重構(gòu)為較小的函數(shù),這些函數(shù)根據(jù)需要被調(diào)用。通常,沒(méi)有必要用tf.function去裝飾那些比較小的函數(shù);僅用tf.function去裝飾高等級(jí)的計(jì)算,例如,訓(xùn)練的一個(gè)步驟,或模型的前向傳遞。
Use Keras layers and models to manage variables
Keras模型和圖層提供了方便variables和 trainable_variables屬性,它以遞歸方式收集所有因變量。這使得在本地管理變量非常容易。
對(duì)比:
def dense(x, W, b):
return tf.nn.sigmoid(tf.matmul(x, W)+ b)
@tf.function
def multilayer_perceptron(x, w0, b0, w1, b1, w2, b2 ...):
x = dense(x, w0, b0)
x = dense(x, w1, b1)
x = dense(x, w2, b2)
...
# 你仍然需要管理w_i和b_i,它們的形狀遠(yuǎn)離代碼定義。
Keras版本:
# 可以調(diào)用每個(gè)圖層,其簽名等效于 linear(x)
layers =[tf.keras.layers.Dense(hidden_size, activation=tf.nn.sigmoid)for _ in range(n)]
perceptron = tf.keras.Sequential(layers)
# layers[3].trainable_variables => returns [w3, b3]
# perceptron.trainable_variables => returns [w0, b0, ...]
Keras layers/models繼承自tf.train.Checkpointable并集成了@tf.function,這使得直接從Keras對(duì)象導(dǎo)出SavedModels或checkpoint成為可能。您不一定要使用Keras的.fitAPI來(lái)利用這些集成。
這是一個(gè)遷移學(xué)習(xí)的例子,演示了Keras如何輕松收集相關(guān)變量的子集。假設(shè)你正在訓(xùn)練一個(gè)帶有共享主干的多頭模型:
trunk = tf.keras.Sequential([...])
head1 = tf.keras.Sequential([...])
head2 = tf.keras.Sequential([...])
path1 = tf.keras.Sequential([trunk, head1])
path2 = tf.keras.Sequential([trunk, head2])
# Train on primary dataset
for x, y in main_dataset:
with tf.GradientTape()as tape:
prediction = path1(x)
loss = loss_fn_head1(prediction, y)
# Simultaneously optimize trunk and head1 weights.
gradients = tape.gradients(loss, path1.trainable_variables)
optimizer.apply_gradients(gradients, path1.trainable_variables)
# Fine-tune second head, reusing the trunk
for x, y in small_dataset:
with tf.GradientTape()as tape:
prediction = path2(x)
loss = loss_fn_head2(prediction, y)
# Only optimize head2 weights, not trunk weights
gradients = tape.gradients(loss, head2.trainable_variables)
optimizer.apply_gradients(gradients, head2.trainable_variables)
# You can publish just the trunk computation for other people to reuse.
tf.saved_model.save(trunk, output_path)
Combine tf.data.Datasets and @tf.function
在內(nèi)存中迭代擬合訓(xùn)練數(shù)據(jù)時(shí),可以隨意使用常規(guī)的Python迭代。或者,tf.data.Dataset是從硬盤讀取訓(xùn)練數(shù)據(jù)流的最好方法。Datasets是可迭代的(不是迭代器),它可以像在Eager模式下的其他Python迭代一樣工作。您可以通過(guò)用tf.function()包裝代碼來(lái)充分利用數(shù)據(jù)集異步預(yù)取/流功能,這將使用AutoGraph等效的圖操作替換Python的迭代。
@tf.function
def train(model, dataset, optimizer):
for x, y in dataset:
with tf.GradientTape()as tape:
prediction = model(x)
loss = loss_fn(prediction, y)
gradients = tape.gradients(loss, model.trainable_variables)
optimizer.apply_gradients(gradients, model.trainable_variables)
如果您使用Keras .fit()API,則無(wú)需擔(dān)心數(shù)據(jù)集迭代。
model.compile(optimizer=optimizer, loss=loss_fn)
model.fit(dataset)
Take advantage of AutoGraph with Python control flow
AutoGraph提供了一種將依賴于數(shù)據(jù)的控制流轉(zhuǎn)換為等效圖形模式的方法,如tf.cond和tf.while_loop。
數(shù)據(jù)相關(guān)控制流出現(xiàn)的一個(gè)常見(jiàn)位置是序列模型。tf.keras.layers.RNN包裝了一個(gè)RNN cell,允許您既可以靜態(tài)也可以動(dòng)態(tài)的循環(huán)展開(kāi)。為了演示,您可以重新實(shí)現(xiàn)動(dòng)態(tài)展開(kāi),如下所示:
classDynamicRNN(tf.keras.Model):
def __init__(self, rnn_cell):
super(DynamicRNN,self).__init__(self)
self.cell = rnn_cell
def call(self, input_data):
# [batch, time, features] -> [time, batch, features]
input_data = tf.transpose(input_data,[1,0,2])
outputs = tf.TensorArray(tf.float32, input_data.shape[0])
state =self.cell.zero_state(input_data.shape[1], dtype=tf.float32)
for i in tf.range(input_data.shape[0]):
output, state =self.cell(input_data[i], state)
outputs = outputs.write(i, output)
return tf.transpose(outputs.stack(),[1,0,2]), state
有關(guān)AutoGraph功能的更詳細(xì)概述,請(qǐng)參閱指南
Use tf.metrics to aggregate data and tf.summary to log it
要記錄摘要,請(qǐng)使用tf.summary.(scalar|histogram|...)上下文管理器將其重定向到編寫(xiě)器。(如果省略上下文管理器,則不會(huì)發(fā)生任何事情。)與TF 1.x不同,摘要直接發(fā)送給編寫(xiě)器; 沒(méi)有單獨(dú)的“合并”操作,也沒(méi)有單獨(dú)的add_summary()調(diào)用,這意味著step必須在調(diào)用點(diǎn)提供該值。
summary_writer = tf.summary.create_file_writer('/tmp/summaries')
with summary_writer.as_default():
tf.summary.scalar('loss',0.1, step=42)
要在將數(shù)據(jù)記錄為摘要之前聚合數(shù)據(jù),請(qǐng)使用 tf.metrics。Metrics是有狀態(tài)的;它們積累值并在您調(diào)用 .result()時(shí)返回結(jié)果。清除積累值,請(qǐng)使用 .reset_states()。
def train(model, optimizer, dataset, log_freq=10):
avg_loss = tf.keras.metrics.Mean(name='loss', dtype=tf.float32)
for images, labels in dataset:
loss = train_step(model, optimizer, images, labels)
avg_loss.update_state(loss)
if tf.equal(optimizer.iterations % log_freq,0):
tf.summary.scalar('loss', avg_loss.result(), step=optimizer.iterations)
avg_loss.reset_states()
def test(model, test_x, test_y, step_num):
loss = loss_fn(model(test_x), test_y)
tf.summary.scalar('loss', loss, step=step_num)
train_summary_writer = tf.summary.create_file_writer('/tmp/summaries/train')
test_summary_writer = tf.summary.create_file_writer('/tmp/summaries/test')
with train_summary_writer.as_default():
train(model, optimizer, dataset)
with test_summary_writer.as_default():
test(model, test_x, test_y, optimizer.iterations)
通過(guò)將TensorBoard指向摘要日志目錄來(lái)可視化生成的摘要:tensorboard --logdir /tmp/summaries。
-
開(kāi)源
+關(guān)注
關(guān)注
3文章
3123瀏覽量
42064 -
tensorflow
+關(guān)注
關(guān)注
13文章
327瀏覽量
60413
原文標(biāo)題:TensorFlow 2.0高效開(kāi)發(fā)指南
文章出處:【微信號(hào):MachineEpoch,微信公眾號(hào):MachineEpoch】歡迎添加關(guān)注!文章轉(zhuǎn)載請(qǐng)注明出處。
發(fā)布評(píng)論請(qǐng)先 登錄
相關(guān)推薦
USB2.0與OTG規(guī)范及開(kāi)發(fā)指南(全中文)
電子系統(tǒng)設(shè)計(jì)開(kāi)發(fā)板開(kāi)發(fā)指南V2.0
CPLD FPGA高級(jí)應(yīng)用開(kāi)發(fā)指南
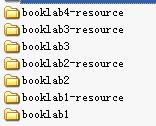
評(píng)論